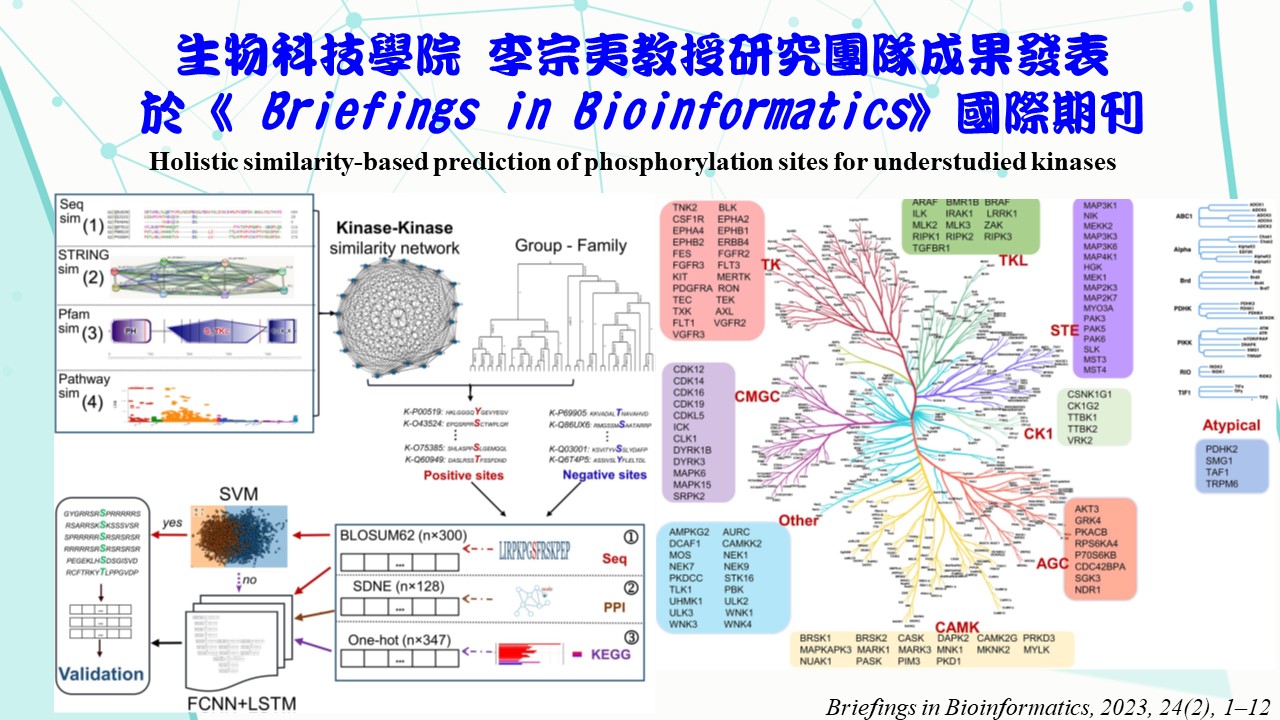
李宗夷教授研究團隊發表研究成果於Briefings in Bioinformatics
連結網址:https://pubmed.ncbi.nlm.nih.gov/36810579/
Abstract
Phosphorylation is an essential mechanism for regulating protein activities. Determining kinase-specific phosphorylation sites by experiments involves time- consuming and expensive analyzes. Although several studies proposed computational methods to model kinase-specific phosphorylation sites, they typically required abundant experimentally verified phosphorylation sites to yield reliable predictions. Nevertheless, the number of experimentally verified phosphorylation sites for most kinases is relatively small, and the targeting phosphorylation sites are still unidentified for some kinases. In fact, there is little research related to these understudied kinases in the literature. Thus, this study aims to create predictive models for these understudied kinases. A kinase-kinase similarity network was generated by merging
the sequence-, functional-, protein-domain- and ‘STRING’-related similarities. Thus, besides sequence data, protein-protein interactions and functional pathways were also considered to aid predictive modelling. This similarity network was then integrated with a classification of kinase groups to yield highly similar kinases to a specific understudied type of kinase. Their experimentally verified phosphorylation sites were leveraged as positive sites to train predictive models. The experimentally verified phosphorylation sites of the understudied kinase were used for validation. Results demonstrate that 82 out of 116 understudied kinases were predicted with adequate performance via the proposed modelling strategy, achieving a balanced accuracy of 0.81, 0.78, 0.84, 0.84, 0.85, 0.82, 0.90, 0.82 and 0.85, for the ‘TK’, ‘Other’, ‘STE’, ‘CAMK’, ‘TKL’, ‘CMGC’, ‘AGC’, ‘CK1’ and ‘Atypical’ groups, respectively. Therefore, this study demonstrates that web-like predictive networks can reliably capture the underlying patterns in such understudied kinases by harnessing relevant sources of similarities to predict their specific phosphorylation sites.